An Automated Approach for Bridging Tool Gaps and Enhancing Performance Metrics
Optimizing Battery Pack Design
Explore how a unified methodology in battery pack design bridges gaps between existing tools, enables efficient optimization of metrics such as energy density, lifespan, safety, and costs.
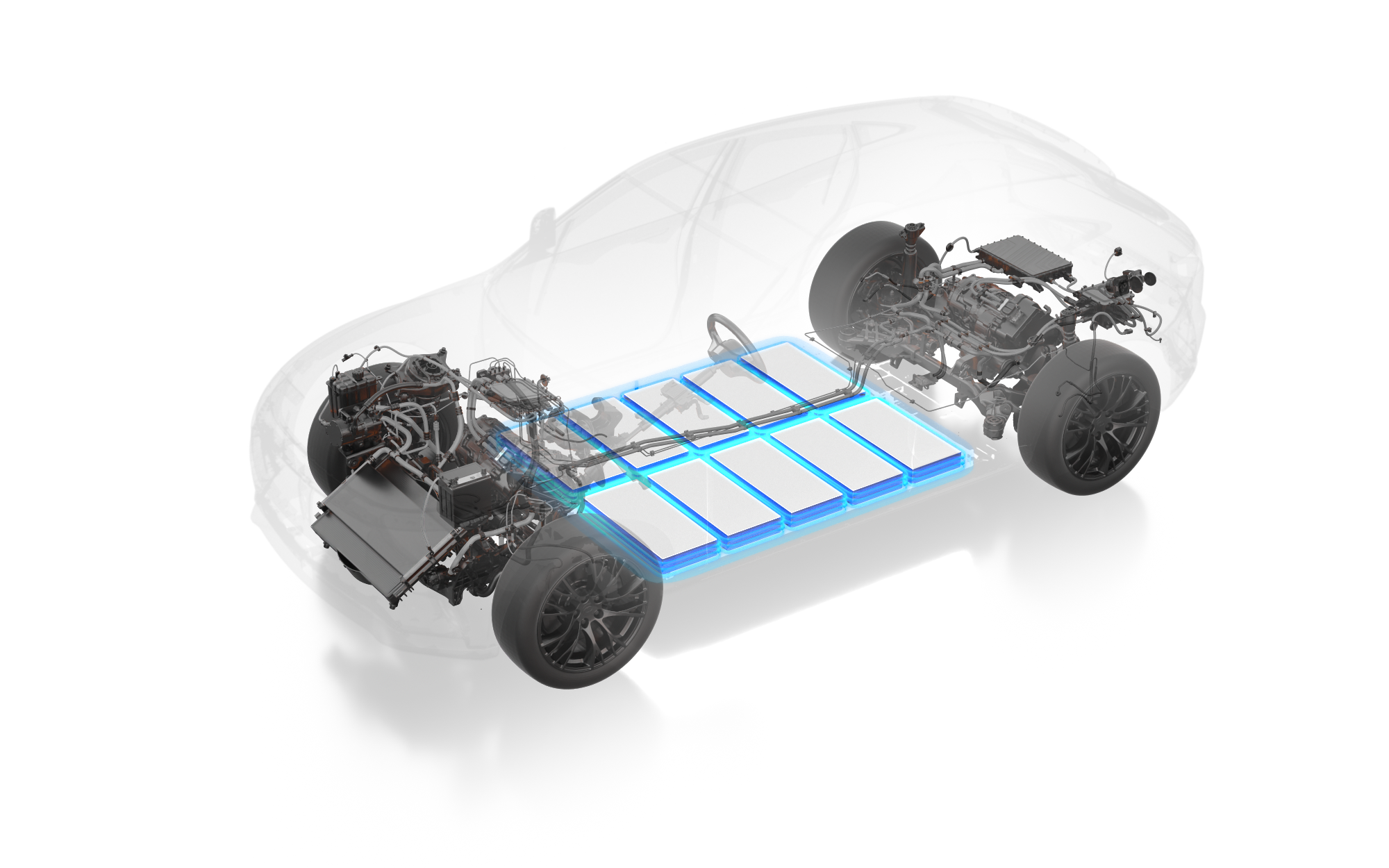
Challenge
Modern battery system engineering operates at the intersection of multiple demanding objectives, with each parameter exerting its own influence on the overall performance and efficiency of the design. Engineers are faced with the formidable challenge of optimizing energy density, quantified as kwh/kg, ensuring longevity in terms of years or operational cycles, maintaining paramount safety standards, and achieving all these within defined cost parameters.
Battery system engineers are presented with the multifaceted task of balancing diverse objectives: energy density (kwh/kg), lifespan (years or cycles), safety, and cost factors. Present toolchains exhibit constraints in addressing these parameters concurrently at multi-scale levels, resulting in fragmented and sequential workflows. CAD tool updates, considering component intricacy, often become time-intensive, thus limiting swift iterative processes. Additionally, design modifications frequently necessitate manual interventions by CAE professionals due to the absence of robust associativity, extending feedback duration.
Solution
Synera is designed as a comprehensive software platform that offers an effective tools stack for the battery pack development, emphasizing integration of design, CAx, and prompt KPI evaluation. In essence, Synera not only simplifies the complex battery design process but accelerates it, driving innovation and ensuring a competitive edge in the marketplace.
Explore the intricacies of our integrated workflows, meticulously engineered to streamline the development process of battery pack design:
- Cell/Block Design Phase: The foundational input is the data from various battery cells. But instead of navigating granular design specifics, the engineer controls the workflow from a strategic viewpoint, delineating constraints and objectives, notably in terms of capacity and voltage Using this framework, a methodical Design of Experiments is initiated, aiming to determine the most suitable block size and configuration. The result is the optimal block geometry.
- Packing Optimization: This stage is dedicated to determining specifications that are later used for the battery module and pack design. It seeks to define the optimal module count and its spatial positioning within the broader battery pack design framework. This phase is enriched by the insights derived from the preceding block geometry. Consequently, a subsequent design experiment is conducted, discerning the optimal block count per module. An advanced nesting algorithm then takes precedence, identifying the optimal packing strategy. The outcome of this phase is a detailed understanding of module dimensions, their specific orientation, and precise positioning within the overall battery pack design space.
- Module Design & Feature Optimization: The workflow delves into a detailed exploration of the module design. Central to this is the integration of a cooling plate, backed by rigorous thermal analysis. Based on the data from the previous phases, the workflow lastly enables the assembly (cells, blocks, cooling plate) of the finished battery module ready to be integrated in the final pack design.
- Pack Design & Structural Optimization: The primary objective is to finalize the pack design. This entails integrating aspects such as structural analysis, lightweight optimization, and the arrangement of the final cable routing. To achieve this, existing data, such as battery pack design parameters and the pre-assembled modules are considered. Based on this foundational data, a series of Finite Element (FE) analyses are conducted. These analyses feature modal evaluations and explore the suitability of various materials, such as aluminum and composites, to provide deeper insights into the optimal material choice. Lightweight design methods, specifically topology optimization, are integrated seamlessly using external tools like Altair OptiStruct. As the design progresses, iterative CAD refinements become essential. These refinements encompass automatic reconstructions based on the results from the topology optimization and a robust parametric design workflow ensuring the design's accuracy and integrity. The Synera internal Path Finder algorithm complements this process, streamlining the intricate task of module cable routing. Upon concluding this phase, a comprehensive overview of Key Performance Indicators (KPIs) is presented, highlighting metrics such as voltage, current, and component weight, and elaborating on the implications of each design decision on these primary metrics.
More about this workflow
Benefits
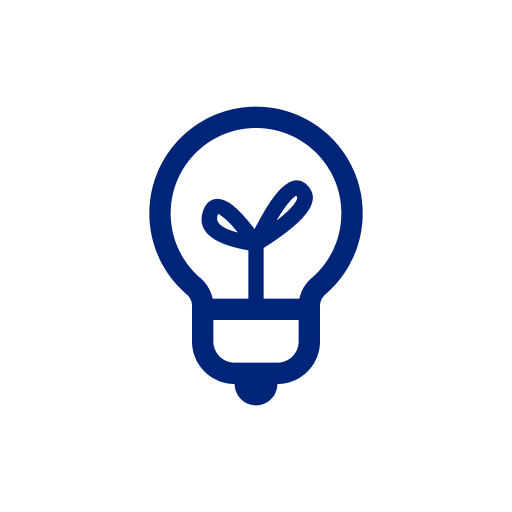
Integrated Design and Analysis
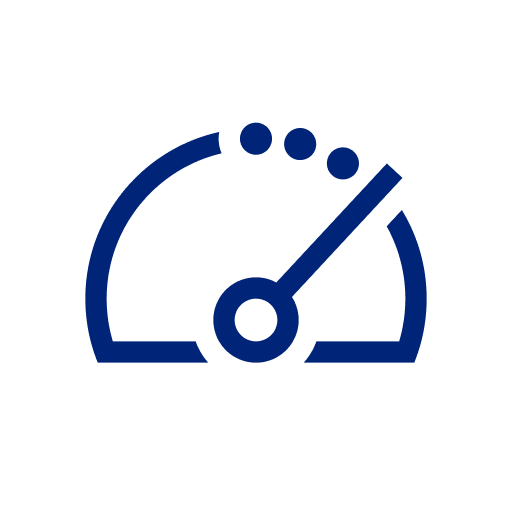
Rapid KPI Evaluation